
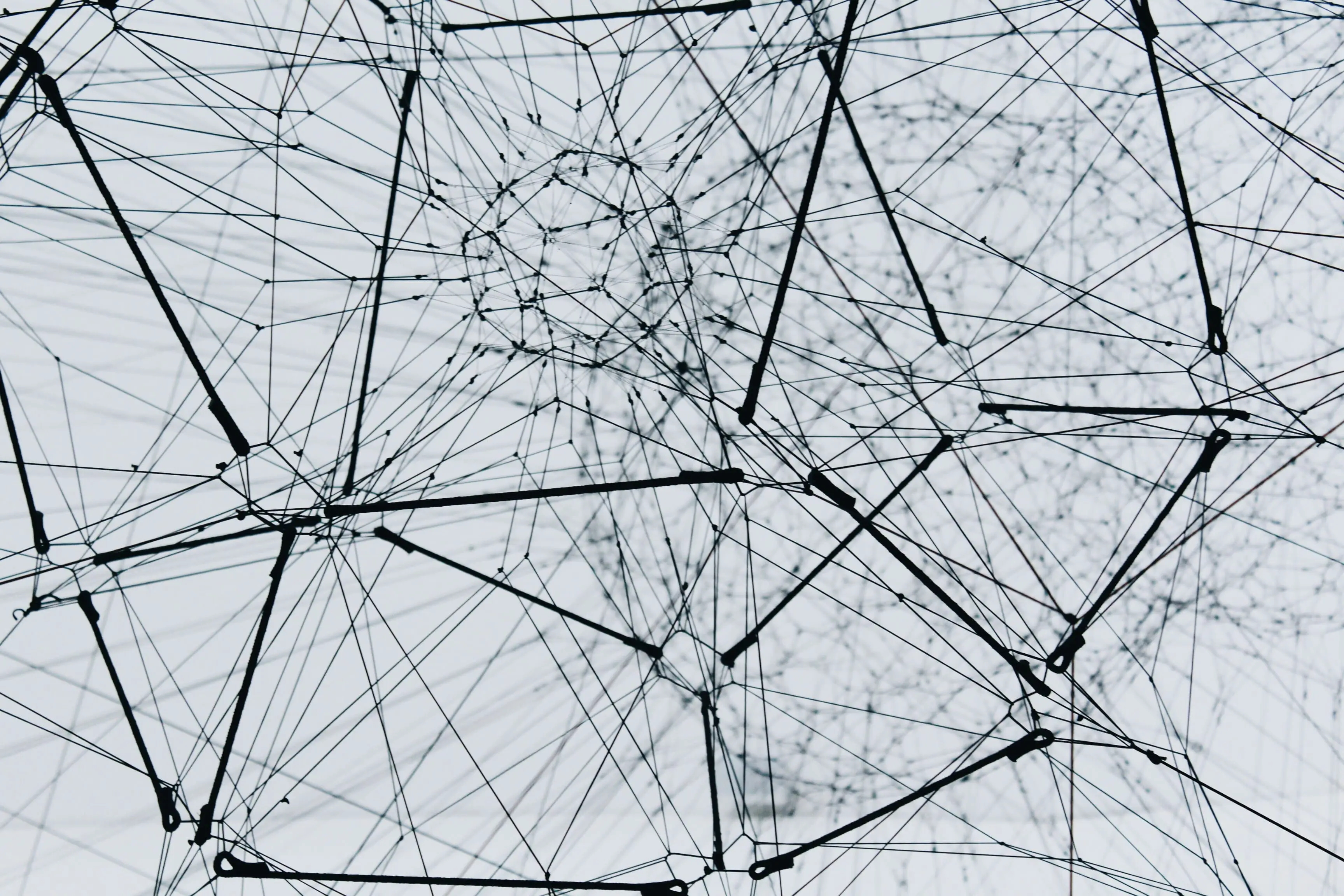
Agent-Based Modeling (ABM) has emerged as a powerful tool in healthcare research, allowing researchers to simulate complex systems at various scales, from cellular interactions to population-level dynamics. This article explores the application of ABM in healthcare, its methodologies, advantages, challenges, and notable contributions to the field.
Introduction to Agent-Based Modeling
Agent-Based Modeling (ABM) is a computational modeling technique used to simulate the actions and interactions of autonomous agents within a defined environment. Each agent follows a set of rules and behaviors, allowing for the exploration of emergent patterns and outcomes that arise from their interactions. In healthcare, these agents can represent anything from individual cells to patients, healthcare providers, or even entire populations [1].
Methodologies and Implementation in Healthcare
ABM begins with defining agents, their behaviors, and the environment in which they operate. For healthcare applications, agents may represent individual patients, pathogens, healthcare facilities, or even policies and interventions [2]. These agents interact based on predefined rules and parameters, simulating realistic scenarios such as disease spread, treatment efficacy, or healthcare resource allocation.
Advantages of ABM in Healthcare Research
1. Complexity and Realism: ABM allows researchers to model complex, nonlinear interactions that are difficult to capture using traditional mathematical or statistical approaches. This realism enables a more accurate representation of healthcare systems and interventions [3].
2. Adaptability and Flexibility: Researchers can easily modify agent behaviors, environmental factors, or intervention strategies within the model to explore various "what-if" scenarios. This adaptability makes ABM suitable for studying dynamic and evolving healthcare challenges [4].
3. Personalized and Population-Level Insights: ABM can simulate both individual patient outcomes and population-level trends simultaneously. This dual focus provides insights into how interventions impact both individuals and communities, supporting personalized medicine and public health decision-making [5].
Applications and Case Studies
Disease Modeling and Epidemiology
ABM has been instrumental in modeling infectious disease dynamics, such as the spread of influenza, HIV/AIDS, and COVID-19. By simulating agent interactions and disease transmission within populations, researchers can evaluate the effectiveness of different intervention strategies, vaccination campaigns, and healthcare policies [6].
Healthcare Policy and Resource Allocation
In healthcare policy, ABM helps policymakers understand the impact of resource allocation decisions on patient outcomes and healthcare system performance. For example, models can simulate the effects of hospital bed capacities, staffing levels, or funding allocations during public health crises or natural disasters [7].
Chronic Disease Management and Treatment Optimization
For chronic diseases like diabetes or cardiovascular conditions, ABM can simulate long-term patient behaviors, treatment adherence, and disease progression. These models aid in optimizing treatment protocols, predicting healthcare utilization, and assessing the cost-effectiveness of preventive interventions [8].
Challenges and Considerations
While powerful, ABM in healthcare research faces several challenges:
1. Data Availability and Validation: Models require accurate data on agent behaviors, disease dynamics, and healthcare system parameters for validation and reliable predictions [9].
2. Complexity and Computational Resources: Simulating large-scale healthcare systems with numerous interacting agents demands significant computational power and expertise in model development and analysis [10].
3. Interdisciplinary Collaboration: Successful ABM implementation often requires collaboration between healthcare experts, epidemiologists, computer scientists, and policy analysts to ensure the model's relevance and applicability [11].
Application of ABM in Apollo2028
In the Apollo2028 project, we will utilize Agent-Based Modeling (ABM) as part of our integrated model definition to analyze the resilience of health and care workers. This integrated model will be developed using data collected from various work packages, focusing on individual, team, and organizational factors. By simulating the interactions between these elements, we aim to understand how feedback loops—such as the influence of individual resilience on team dynamics and, subsequently, on organizational health—affect overall resilience within healthcare systems. The ABM will be data-driven, with its rules derived from empirical datasets, allowing us to provide targeted stakeholders—such as health managers and policymakers—with actionable insights to better manage workforce resilience. The model will be shared as a core deliverable, offering vital knowledge to improve healthcare management strategies.
Future Directions and Conclusion
Looking ahead, ABM holds promise for advancing healthcare research by integrating more granular data, improving model accuracy, and exploring new applications in personalized medicine, health equity, and global health security. As computational capabilities evolve and interdisciplinary collaborations deepen, ABM will continue to play a pivotal role in shaping evidence-based healthcare policies and practices worldwide [12].
References
1. Bonabeau, E. (2002). Agent-based modeling: Methods and techniques for simulating human systems. *Proceedings of the National Academy of Sciences*, 99(Suppl 3), 7280-7287. doi:10.1073/pnas.082082699
2. Macal, C. M., & North, M. J. (2005). Agent-based modeling and simulation: ABMS. *Wiley Encyclopedia of Operations Research and Management Science*. doi:10.1002/9780470400593.eorms0568
3. Grimm, V., & Railsback, S. F. (2005). Individual-based modeling and ecology. *Princeton University Press*.
4. An, L., & Liu, J. (2010). Agent-based modeling for sustainability. *Ecological Complexity*, 7(3), 193-204. doi:10.1016/j.ecocom.2010.01.001
5. Jager, W., & Janssen, M. A. (2002). Modelling human behaviour in ecological economics. *Ecological Economics*, 41(3), 373-392. doi:10.1016/S0921-8009(02)00171-5
6. Vespignani, A. (2009). Predicting the behavior of techno-social systems. *Science*, 325(5939), 425-428. doi:10.1126/science.1172458
7. Yang, Y., & Ehlers, D. (2021). Agent-based modeling of healthcare systems: A literature review. *Health Systems*, 10(1), 1-17. doi:10.1080/20476965.2020.1861666
8. Kuhl, M. E., & Giacalone, R. (2018). Modeling and analysis of chronic disease management: A review of agent-based modeling approaches. *Computational and Mathematical Organization Theory*, 24(1), 1-22. doi:10.1007/s10588-017-9265-4
9. Zhang, J., & O’Neill, J. (2020). Data-driven agent-based models in healthcare. *Health Informatics Journal*, 26(3), 1579-1590. doi:10.1177/1460458218790167
10. Keeling, M. J., & Rohani, P. (2008). Modeling infectious diseases in humans and animals. *Princeton University Press*.
11. Rvachew, S., & G. Wang, Y. (2022). Interdisciplinary collaboration in healthcare simulation: Challenges and opportunities. *Journal of Health Management*, 24(1), 69-85. doi:10.1177/09720634211017062
12. Brailsford, S. C., & Vissers, J. (2011). Modelling in healthcare: A review of the literature. *Journal of the Operational Research Society*, 62(9), 1627-1636. doi:10.1057/jors.2010.51